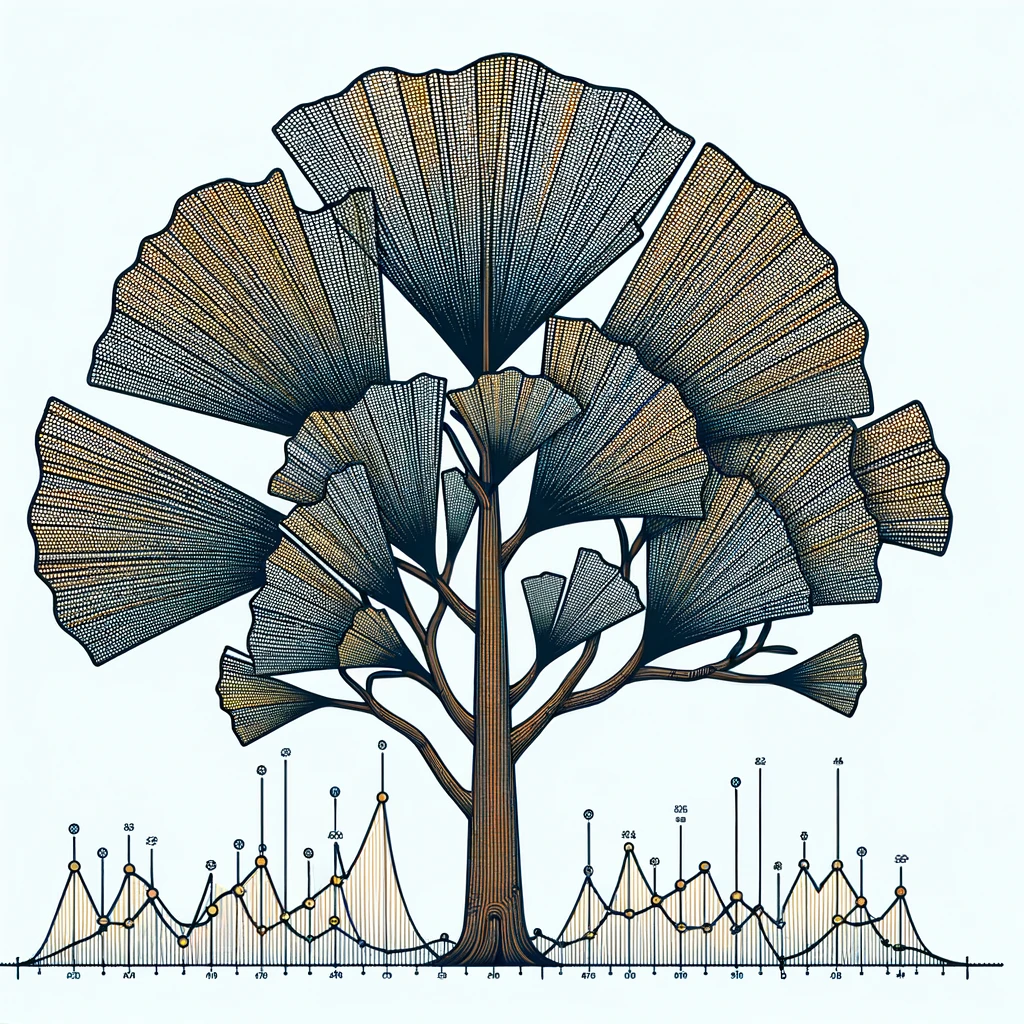
Jupyter Notebooks as Test Cases
Preface
This post is about testing Jupyter Notebooks. If you’re using Notebooks and not well testing your Notebooks, read on!
This post is not highly technical. There are a few technical steps, but the code involved is relatively simple: less than 30 lines of code for Pytest, a configuration file, an environment variable, and a script.
Testing and Jupyter
Jupyter Notebooks are often very critical units of code, and are very often incompletely tested (or not tested at all). Manual testing of Notebooks, or wait till someone complains - the “ship first” mentality - doesn’t scale. Over time, the inventory of Notebooks grows and your development velocity slows to a crawl.
We use Notebooks for multiple purposes. Many Notebooks are Production: they need to run successfully and to completion. We need to know early if any code changes break any Production notebooks or change their behavior. Some Notebooks are development tools that don’t affect end-users but are used occasionally by developers: it’s annoying when you need to use a tool that you have to first fix. And, importantly, some Notebooks serve as test cases: there are a lot of functions where we want to exercise our UI libraries and ensure that the nbappinator
application framework is stable.
In short, you might want to test Notebooks because they’re important (don’t break production) or simply use Notebooks as test cases to verify functional behavior.
This post covers three approaches to Notebook testing:
- Verifying Notebook Completion w/ pytest and nbconvert
- Measuring Notebook Coverage w/ coverage.py
- Detecting Content Changes
I’ll close with a few additional topics:
- Parallel Testing w/ pytest-xdist
- Testing Interactive Behavior
- pre-commit hooks
- Coverage Badges
Code Coverage
Part of this discussion is measuring the code coverage of your Notebooks, whether the Notebooks are production or test cases. Code coverage is a process of identifying which lines of code are reached by some code. It’s commonly used to assess how “complete” test cases1 are: whether the test cases fully exercise all of the code in a repository.
Coverage also can serve as a quality canary in the coal mine. Low or dropping coverage isn’t a problem in and of itself, but it is a signal of potential quality problems.
This post covers how we use coverage.py to measure code coverage of our Notebooks, which helps us:
- Measure how well our Notebook test cases exercise our code: it shows us any code that is not tested by a Notebook (and/or another test case)
- Identify dead code that’s never reached by Production Notebooks 2
- (rarely) Understand which code a particular notebook uses.
Coverage reports help inform our development process. Coverage gaps might result in:
- Writing more or better test cases
- Pruning unused or otherwise dead code
- Identifying difficult-to-test code that must be manually verified (for now)
coverage.py works well with pytest
tests. It requires a little configuration to work with Notebooks, since each Notebook runs in a separate Python kernel. With the following configuration and code, coverage.py will generate a separate .coverage.XYZ file for each notebook. These coverage files are then combined into a single coverage file, and a coverage report is generated.
Technical Note
Pytest
These tests use pytest
. You’ll see examples in https://github.com/iqmo-org/nbappinator/blob/main/tests
. To run our tests, if you like:
- create a virtual environment using your environment manager.
- Open a terminal, mkdir a base directory to clone into, clone the repo:
git clone https://github.com/iqmo-org/nbappinator
cd nbappinator
- Install dependencies:
pip install -r requirements_dev.txt
pytest -n auto
Verifying Notebook Completion
The first, most important, test is: Do all the Notebooks run to completion without an unhandled Exception?
Code
This test case verifies that all notebooks in a directory run to completion with no unhandled exceptions. Behavior is not verified, only that the notebooks finished. This is a good starting point to make sure code changes don’t break the Notebooks.
The test here uses two features:
@pytest.mark.parametrize
to run the test case for every notebook in a directory. You could use a for loop inside the test, but this allows us to usepytest-xdist
to parallelize the tests.
Our implementation is in test_nb.py.
This is a minimal example of merely testing execution to completion. There’s a 600 second (5 min) timeout: adjust to your preferences.
import pytest
from pathlib import Path
import nbformat
from nbconvert.preprocessors import ExecutePreprocessor
NOTEBOOK_DIR = Path("./notebooks")
SKIP_NOTEBOOKS = [] # ["1_readme_example.ipynb"]
TIMEOUT = 600
@pytest.mark.parametrize(
"notebook", [f for f in NOTEBOOK_DIR.glob('**/*.ipynb') if f.name not in SKIP_NOTEBOOKS]
)
def test_notebook_execution(notebook: Path):
with open(notebook) as f:
nb = nbformat.read(f, as_version=4)
ep = ExecutePreprocessor(timeout=TIMEOUT)
ep.preprocess(nb)
Measuring Notebook Coverage
Configuration
Our method to test Notebook coverage uses coverage’s subprocess instructions, requiring three steps:
- Creating a .coveragerc file with parallel=true and omitting ipykernel files. For example: .coveragerc
- Set the COVERAGE_PROCESS_START environment variable, pointing to the .coveragerc
- We use a separate test environment with this environment variable preset. Use whatever virtual env you want: venv/virtualenv/conda/…
- Injecting two lines into each notebook at runtime.
import coverage
coverage.process_startup()
Alternatively, add os.environ["COVERAGE_PROCESS_START"] = 'yourpath/.coveragerc'
in the injected code and skip setting variable in your environment.
Test Case
Our implementation is in test_nb.py.
The only difference between this and the Basic Test code is the injection via nb.cells.insert
:
import pytest
from pathlib import Path
import nbformat
from nbconvert.preprocessors import ExecutePreprocessor
NOTEBOOK_DIR = Path("./notebooks")
SKIP_NOTEBOOKS = []
TIMEOUT = 600
@pytest.mark.parametrize(
"notebook",
[
f for f in NOTEBOOK_DIR.glob('**/*.ipynb') if f.name not in SKIP_NOTEBOOKS
]
)
def test_notebook_execution(notebook: Path, coverage: bool = True):
with open(notebook) as f:
nb = nbformat.read(f, as_version=4)
if coverage:
nb.cells.insert(
0, nbformat.v4.new_code_cell("import coverage\ncoverage.process_startup()")
)
ep = ExecutePreprocessor(timeout=TIMEOUT)
ep.preprocess(nb)
Testing Notes
Using pytest-cov
, much of this process should be automatic as it injects the process_startup
via a .pth file. For an undetermined reason, this was yielding inconsistent results and the above injection was needed regardless.
The above injection also works outside a pytest fixture: an alternative method would be to call the above function for each Notebook.
Running Locally
- In our dev environment, we create a virtual-env/venv, set the environment variable, and run the following tasks (as a script):
# Requires: coverage pytest-cov pytest-xdist nbconvert nbformat
coverage erase
pytest --cov=nbappinator -n auto
coverage combine
coverage report -m
Explanation:
coverage erase
: Clears any existing .coverage filespytest --cov=nbappinator -n auto
:--cov==nbappinator
activates the pytest-cov plugin and designates the package to measure coverage of, which initializes the coverage runner.-n auto
uses pytest-xdist to distribute the tests across multiple processes, with one worker per core.coverage combine
: Combines the intermediate .coverage results from sub-processescoverage report -m
: Generates a coverage report.
Running as a GitHub Action workflow:
- name: Run tests with coverage
env:
COVERAGE_PROCESS_START: ${{ github.workspace }}/.coveragerc
run: |
pytest --cov=nbappinator -n auto
coverage combine
coverage report
Detecting Content Changes
The previous tests only verify that the Notebook ran without error. Any behavior must be checked / asserted in the notebook itself. Assertions can be built into the notebook to verify the behavior, as well.
A different strategy is to establish a baseline, and then compare subsequent runs against that baseline. This is known as snapshot testing, popularized by frameworks like jestjs.
Snapshot testing has pros and cons. Small changes can have rippling affects which make it hard for a developer to know the scope of their changes. Broadly, detecting output changes is a helpful in a release cycle to know which Notebooks deserve a closer manual test.
Our approach to snapshot testing is HTML-centric:
- Store a baseline of the Notebook, usually in an external S3 bucket: keeping code separate from data
- During development, generate a local checkpoint and compare it against the baseline.
- Review any changes
- As part of a release cycle, manually verify any notebooks that have changed: update baseline if appropriate
This is challenging to get right when applied to notebooks with rich/complex HTML: pages that are dynamically generated will have a variety of small changes. Some changes can be resolved through patterns & substitutions, such as run-time generated UUIDs. Some changes can be generally ignore. And, other changes, such as compressed data structures, will confound certain tests.
Regardless, this can be helpful in identifying Notebooks that require a closer look during a release gate. Using simple, single-purpose test notebooks can help. Notebooks that don’t have a lot going on are easier to compare. With Jupyter, some widgets are more problematic than others.
Interactive Content
While snapshot testing is fine for testing code behavior, it’s limited in testing the Javascript interactions. We’ve tried a few approaches at trying to execute arbitrary Javascript, but ultimately had to settle with simulating the Javascript events on the Python side. This requires a longer conversation about how asynchronous events are executed and how Jupyter comms work.
In short, if you truly want to test the interactive content, then you’d likely need to follow a different testing strategy: traditional web-testing frameworks against a Voila deployment of the Notebook.
Generating baselines and comparing
There are a few approaches to comparing notebooks. One is to compare the .ipynb files directly since they’re structured .json files. Alternatively, you could render the Notebooks as .html or .md, and compare the rendered Notebooks.
We choose to compare .html versions of the notebooks. This fit well into process of only committing code-only notebooks. Rich web components are also difficult to compare, and there are many tools for comparing / differencing html files. It also made it easy to inspect the notebooks offline with only a web browser, even though there’d be no backend kernel running.
There’s a functioning version of this in our nbappinator project. The idea is that you can generate the baseline (once) with: python -m tests.snapshot_mgr --generate_baseline
. During development, you can run: python -m tests.snapshot_mgr --generate
and then python -m tests.snapshot_mgr --compare
to compare the most recent checkpoint against the baseline. Any changed files are shown. python -m tests.snapshot_mgr --compare --report
shows a report of the changes. This version supports using an s3 repository for the baseline, using universal-pathlib and s3fs.
This comparison does a few things to make the HTML more easily comparable: prettifying the HTML and embedded JSON, replacing UUIDs with deterministic identifiers, stripping trailing/leading whitespace from lines, and ignoring other lines. This was sufficient for our purposes, but any HTML comparison utility may work here.
Additional Topics
Use pytest-xdist
pytest-xdist extends pytest with parallel workers. This is very helpful when running Notebooks that already run in separate kernels and are long-running tests. Using pytest is as simple as:
pip install pytest-xdist
pytest -n auto
pre-commit hooks
pre-commit is a framework to run code (“hooks”) before every commit to a git repository. There are many types of pre-commit hooks. For Notebooks, the two important pre-commits are:
- nbstripout: removes data from notebooks, ensuring that our commits are code-only notebooks.
- ruff: lints and formats Jupyter notebooks, following standard Python conventions.
- pytest: runs test cases before each commit.
In many repositories, it’s not practical for a developer to run every test locally, but it is possible to have them run some tests to catch obvious breaks. Instead, a GitHub Action workflow (or other CI tool) is used to run tests. Running a pytest pre-commit is mainly to catch the obvious mistakes.
Pre-commits have to be installed by each developer: developers can easily bypass the pre-commits. They’re just a way to keep the git repo somewhat stable.
We also use a variety of other pre-commits for our code-base, including: linters, type checkers, and code formatters.
.pre-commit-config.yaml pytest example
An example pre-commit that runs pytest against tests in the tests
directory whenever .py or .ipynb files are modified.
- repo: local
hooks:
- id: pytest
name: pytest
entry: pytest -n auto tests
language: system
types: [python]
pass_filenames: false
always_run: false
files: '.*\.(py|ipynb)$'
Coverage Badges
A coverage badge in a README.md file (or another file) is a good way to prioritize coverage: it’s a reminder to do better each release.
Understanding your coverage is the goal. No more, no less. 100% coverage of all lines of code is not a necessary goal: you can have 100% coverage with poor tests, or low coverage that adequately verifies critical business logic. The latter (low but sufficient) could be redefined as 100% coverage of code that matters.
This is done in our GitHub Action workflow, using python-coverage-comment-action
- name: Python Coverage Comment
uses: py-cov-action/python-coverage-comment-action@v3.23
with:
GITHUB_TOKEN: ${{ github.token }}
Other Tools
Packages we evaluated but ultimately didn’t use:
- nbmake: A good tool with some good features, such as tags to exclude certain notebooks and to skip long-running cells. It didn’t solve any problems we had right now, but it is something we might re-evaluate.
- nbval: Runs and compares notebooks against their last execution. This didn’t work well with our rich HTML notebooks, where rendering them to HTML allows us to use general web-testing/differencing tools.
Footnotes
-
Code coverage doesn’t have to be restricted to test cases; you could also test some code execution for other purposes. ↩
-
This isn’t a perfect evaluation, since some code paths depend on inputs that production notebooks don’t hit when run statically, but it’s a helpful tool in identifying code that might be prunable. ↩